Keynote Speakers
Dr. Pascale Fung
Professor at The Hong Kong University of Science & Technology
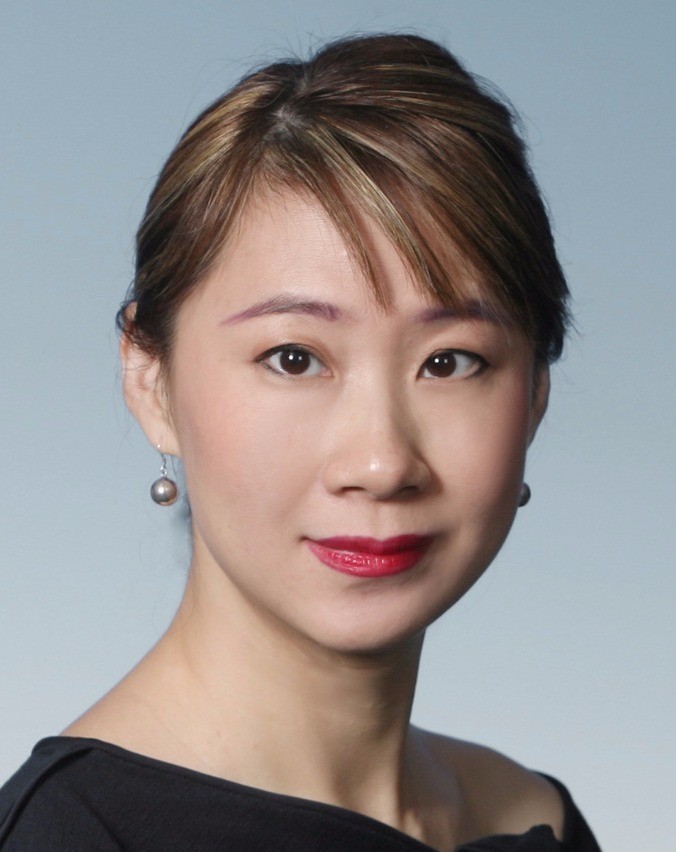
Talk title: "Conversational AI"
Conversational AI systems interact with human users while completing user requests or simply chit-chat. These systems have applications ranging from personal assistance, health assistance to customer services, etc. In this three-part talk, we will first give an overview of the state-of-the-art modularized conversational AI approaches that are commonly adopted by task-oriented dialog systems. We will then give an overview of the current sequence to sequence , generation-based conversational AI approaches. We will discuss the challenges and shortcomings of vanilla generation-based models such as the lack of knowledge, consistency, empathy, controllability, versatility, etc. We will then highlight current work in addressing these challenges and in improving the depth of generation-based ConvAI. In the final part of the talk we will point out remaining challenges of conversational AI and possible directions for future research, including how to mitigate inappropriate responses and lifelong learning. We will also present an overview of shared tasks and publicly available resources for both modularized and generation-based conversational AI.Dr. Christopher Manning
Professor at Stanford University
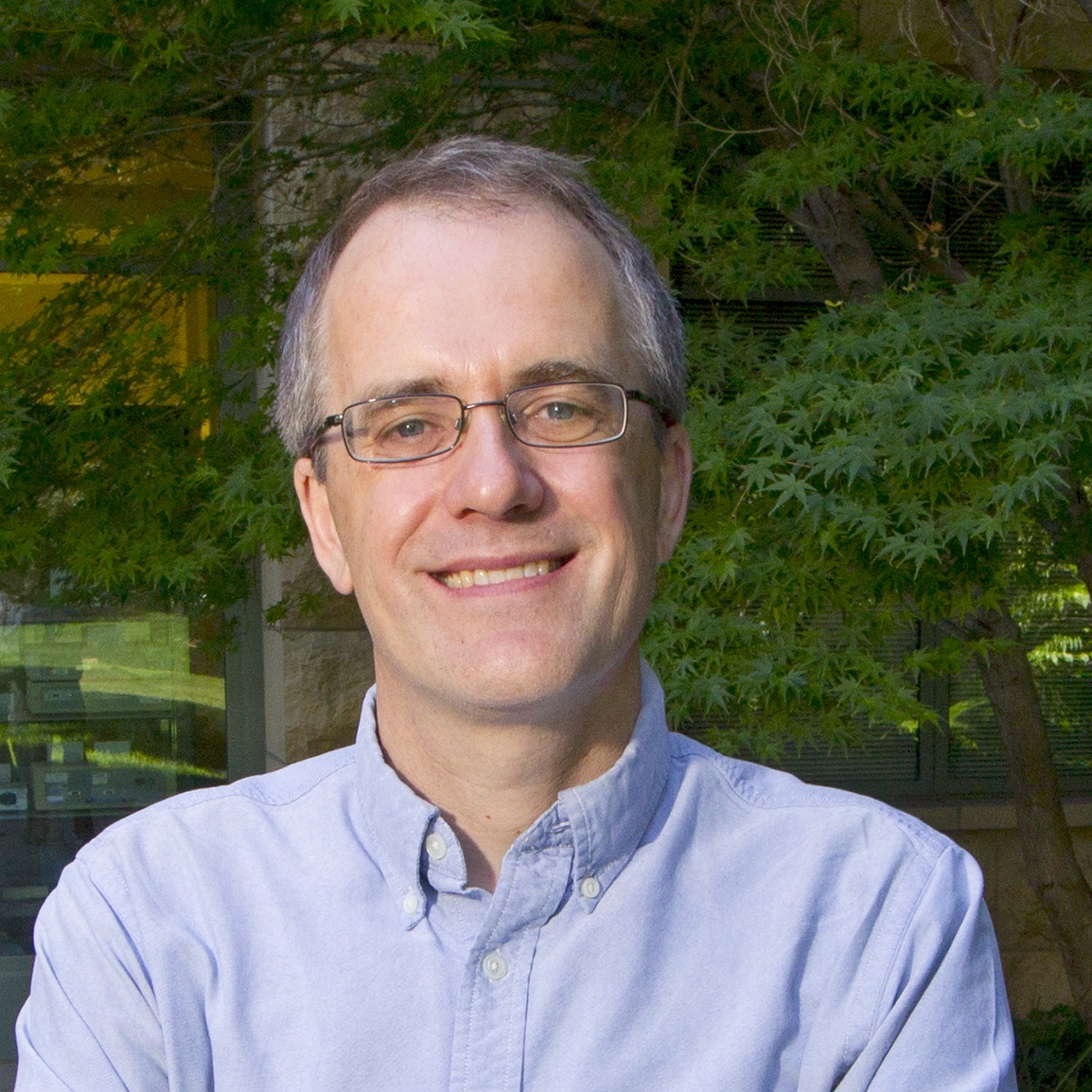
Talk title: "Large Language Models: Linguistic Structure Discovery and More Efficient Training"
In this talk, I will first briefly outline the recent sea change in NLP with the rise of large pre-trained transformer language models, such as BERT, and the effectiveness of these models on NLP tasks. I will then focus in on two particular aspects. First, I will show how, despite only using a simple self-supervision task, BERT-like models not only capture word associations but act as linguistic structure discovery devices, capturing such things as human language syntax and pronominal coreference. Secondly, I will emphasize how recent progress has been bought at enormous computational cost and explore the ELECTRA model, in which an alternative discriminative learning method allows building highly effective neural word representations with considerably less computation.Dr. Rada Mihalcea
Professor at the University of Michigan
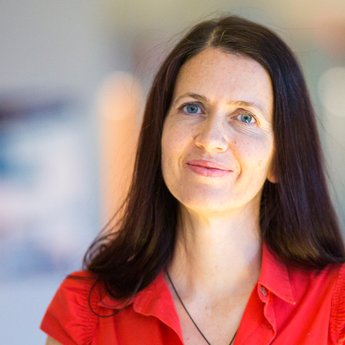
Talk title: "The Other Side(s) of Word Embeddings"
Word embeddings have largely been a "success story" in our field. They have enabled progress in numerous language processing applications, and have facilitated the application of large-scale language analyses in other domains, such as social sciences and humanities. While less talked about, word embeddings also have many shortcomings -- instability, lack of transparency, biases, and more. In this talk, I will review the "ups" and "downs" of word embeddings, discuss tradeoffs, and chart potential future research directions to address some of the downsides of these word representations.Dr. Sujith Ravi
Director at Amazon Alexa AI
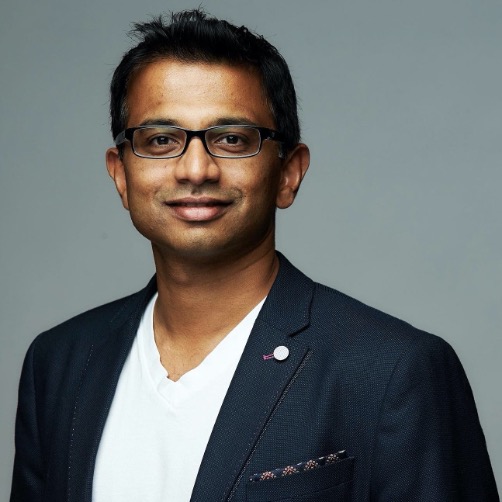
Dr. Ravi has authored over 100 scientific publications and patents in top-tier machine learning and natural language processing conferences. His work has been featured in press: Wired, Forbes, Forrester, New York Times, TechCrunch, VentureBeat, Engadget, New Scientist, among others, and also won the SIGDIAL Best Paper Award in 2019 and ACM SIGKDD Best Research Paper Award in 2014. For multiple years, he was a mentor for Google Launchpad startups. Dr. Ravi was the Co-Chair (AI and deep learning) for the 2019 National Academy of Engineering (NAE) Frontiers of Engineering symposium. He was also the Co-Chair for ACL 2021, EMNLP 2020, ICML 2019, NAACL 2019, and NeurIPS 2018 ML workshops and regularly serves as Senior/Area Chair and PC of top-tier machine learning and natural language processing conferences like NeurIPS, ICML, ACL, NAACL, AAAI, EMNLP, COLING, KDD, and WSDM.
Talk title: "Powering Deep Learning with Structure"
Deep learning advances have enabled us to build high-capacity intelligent systems capable of perceiving and understanding the real world from text, speech and images. Yet, building real-world, scalable intelligent systems from “scratch” remains a daunting challenge as it requires us to deal with ambiguity, data sparsity and solve complex language & visual, dialog and generation problems. In this talk, I will present powerful neural structured learning frameworks, precursor to widely-popular GNNs, that tackle the above challenges by leveraging the power of deep learning combined with graphs which allow us to model the structure inherent in language and visual data. We use graph-based machine learning as a computing mechanism to design efficient algorithms and address these challenges. Our neural graph learning approach handles massive graphs with billions of vertices and trillions of edges and has been successfully used to power real-world applications at industry scale for response generation, image recognition and multimodal experiences. I will highlight our work on using neural graph learning with a novel class of attention mechanisms over Euclidean and Hyperbolic spaces to model complex patterns in Knowledge Graphs for learning entity relationships, predicting missing facts and performing multi-hop reasoning. Finally, I will describe recent work on leveraging graphs for multi-document news summarization.Contact information
- LinkedIn: Dr. Ravi's LinkedIn
- Web: Personal page.
- Twitter: @ravisujith
Dr. Heng Ji
Professor at the University of Illinois at Urbana-Champaign
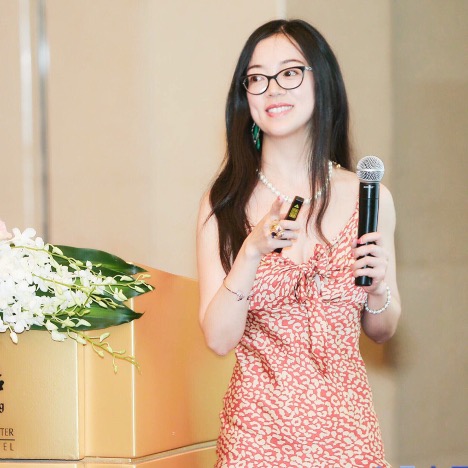
Talk title: "How to Write a History Book?"
Understanding events and communicating about events are fundamental human activities. However, it's much more difficult to remember event-related information compared to entity-related information. For example, most people in Mexico will be able to answer the question "Which city is Universidad Nacional Autónoma de México is located in?", but very few people can give a complete answer to "Who died from COVID-19?". Human-written history books are often incomplete and highly biased because "History is written by the victors". In this talk I will present a new research direction on event-centric knowledge base construction from multimedia multilingual sources, and then perform consistency checking and reasoning to detect and correct misinformation. Our minds represent events at various levels of granularity and abstraction, which allows us to quickly access and reason about old and new scenarios. Progress in natural language understanding and computer vision has helped automate some parts of event understanding but the current, first-generation, automated event understanding is overly simplistic since it is local, sequential and flat. Real events are hierarchical and probabilistic. Understanding them requires knowledge in the form of a repository of abstracted event schemas (complex event templates), understanding the progress of time, using background knowledge, and performing global inference. Our approach to second-generation event understanding builds on an incidental supervision approach to inducing an event schema repository that is probabilistic, hierarchically organized and semantically coherent. This facilitates inducing higher-level event representations analysts can interact with, and allow them to guide further reasoning and extract events by constructing a novel structured cross-media cross-lingual common semantic space. To understand the many facets of such complex, dynamic situations, we have developed various novel methods to induce hierarchical narrative graph schemas and apply them to enhance end-to-end joint neural Information Extraction, event coreference resolution, event time prediction, and misinformation detection.Dr. Sebastian Ruder
Researcher at DeepMind
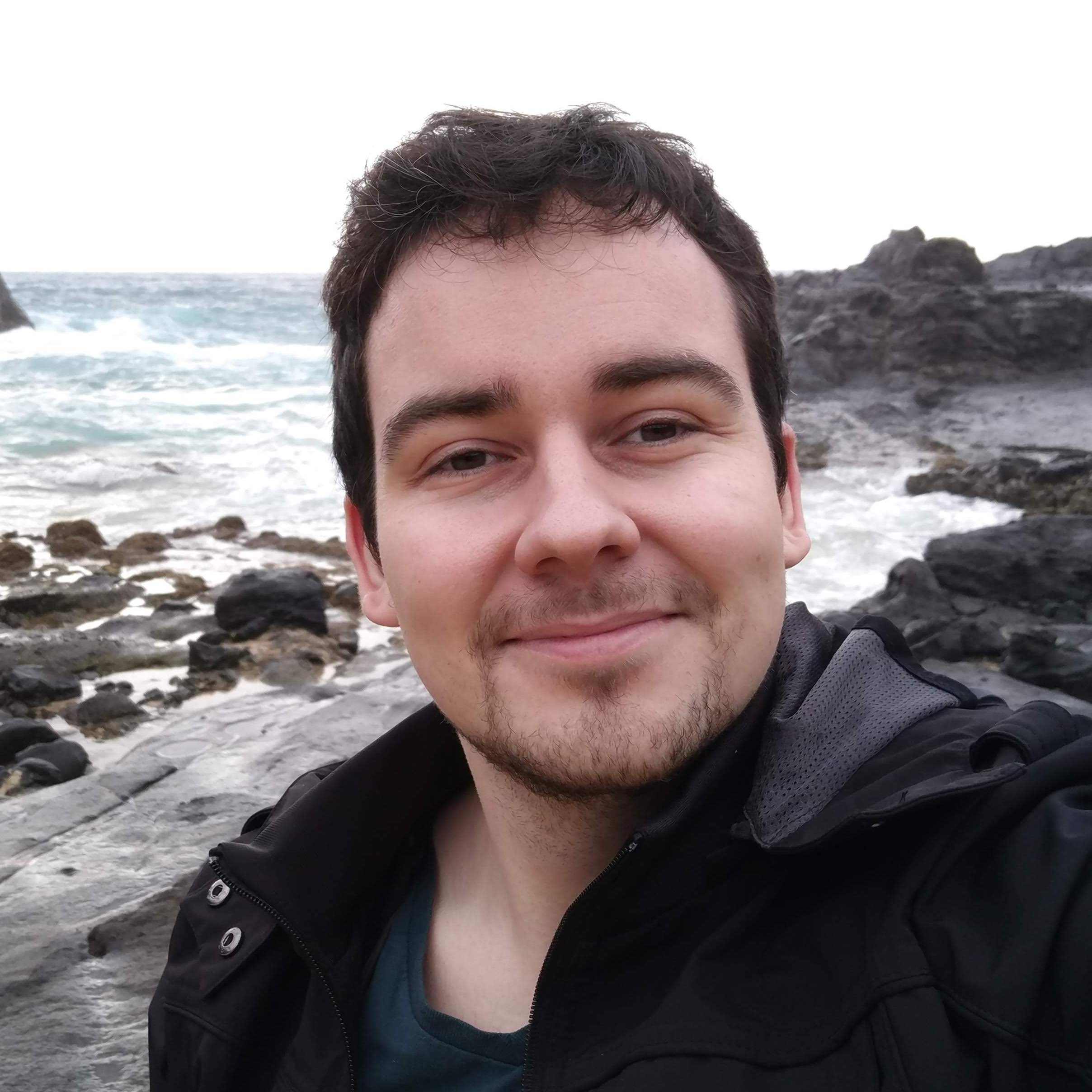
Talk title: "Challenges in Cross-lingual Transfer Learning"
Research in natural language processing (NLP) has seen striking advances in recent years but most of this success has focused on English. In this talk, I will give an overview of approaches that transfer knowledge across languages and enable us to scale NLP models to more of the world's 7,000 languages. I will cover open challenges in this area such as evaluation in the face of limited labelled data, generalizing to low-resource languages and different scripts, and dealing with erroneous segmentations and discuss approaches that help mitigate them.Contact information
- Web: Personal page.
- Twitter: @seb_ruder
Tutorials
Dr. Tanja Samardžić
Lecturer at University of Zurich, Switzerland
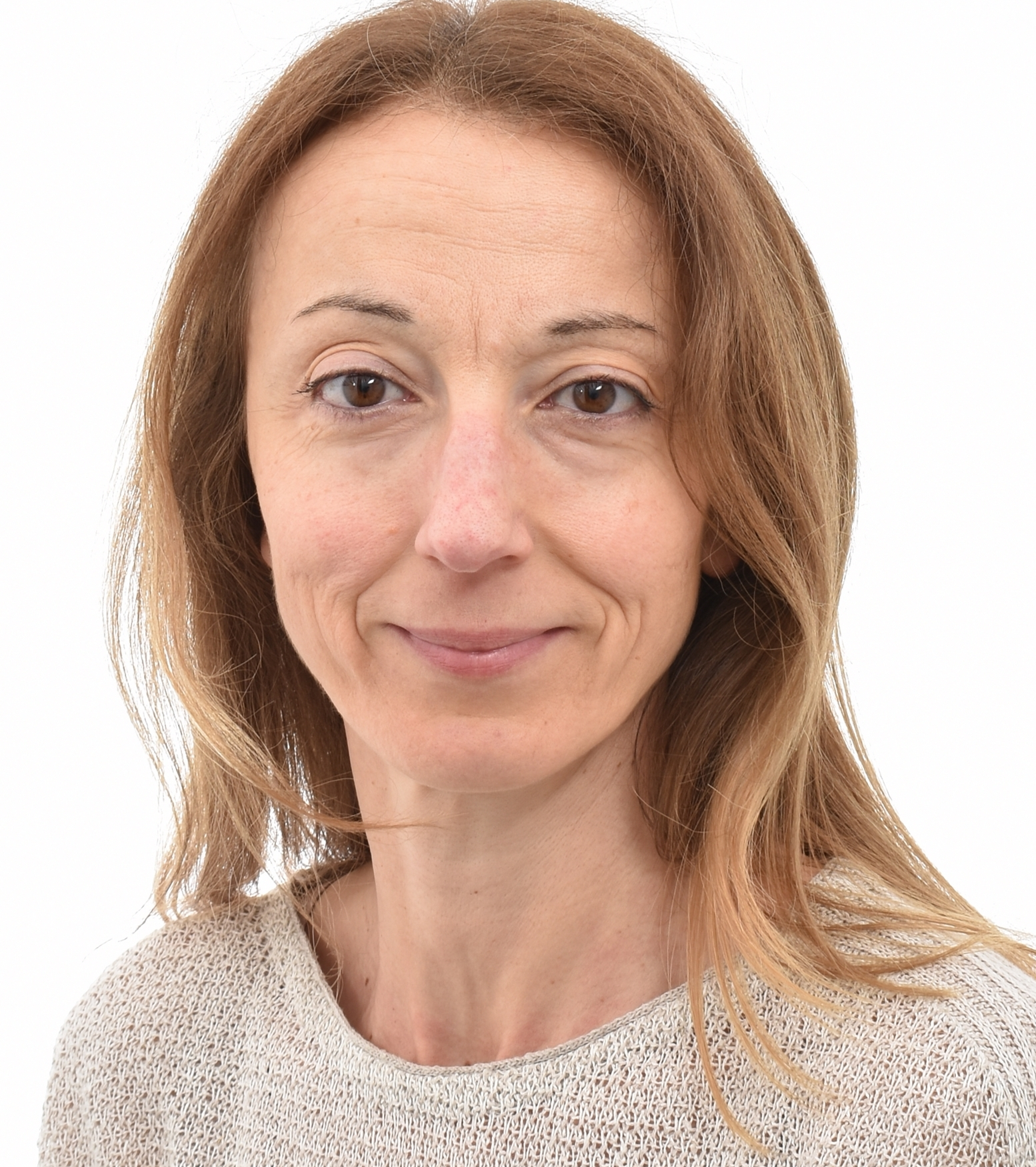
Tutorial: “Language (de)standardisation and NLP”
The term non-standard language covers all linguistic expressions not conforming to an official orthography and pronunciation. Such expressions include dialects (written and spoken), historical texts and social media posts. They require special processing techniques in order to deal with the fact that the same word can be written (or pronounced) very differently in the same text (or recording). This tutorial will provide an introduction to the notion of language standard and an overview of the challenges that increasingly non-standard writing and speech pose to NLP. We will try out text normalisation as a technique to deal with noisy text and discuss other potential approaches.Contact information
- Web: Personal page.
Dr. Alexander Gelbukh y Dr. Grigori Sidorov
Full Professors at CIC-IPN, Mexico
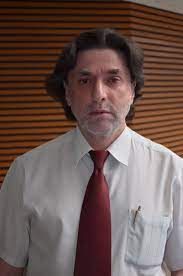
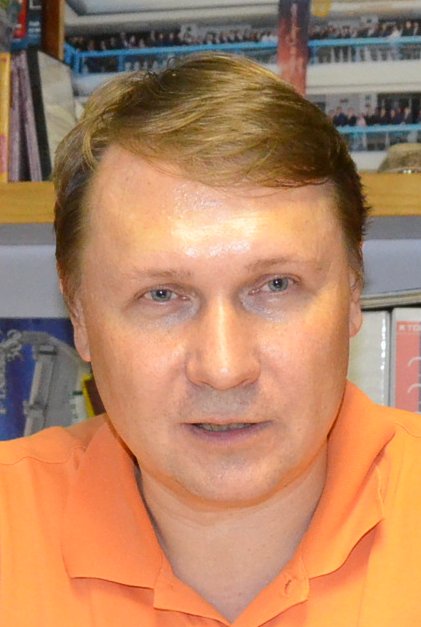
Tutorial: Natural Language Processing, Introduction
In the first part of the tutorial, the motivation behind the study of natural language processing will be discussed. Some of its applications will be presented, the dangers that the development of this area entails (and from which we must learn to defend ourselves), as well as in a very brief way some methods that are used. Finally, an invitation will be made to study a Master or PhD (with full scholarship) in this area. In the second part, general ideas related to the field of artificial intelligence will be presented. The vector space model, cosine similarity, feature selection and types of features (bag of words, n-grams of various types, syntactic n-grams) and the values of the features (tf, tf-idf) will be briefly described. The application of machine learning methods to the analysis of texts will be presented. Various applications will be conceptually described: for example, how the author profiling (gender, age), spam detection, and authorship attribution, among others, can be automatically performed.Contact information
- Web: Alexander Gelbukh
- Web: Grigori Sidorov
Dr. Leticia C. Cagnina
Professor at the Universidad Nacional de San Luis, Argentina
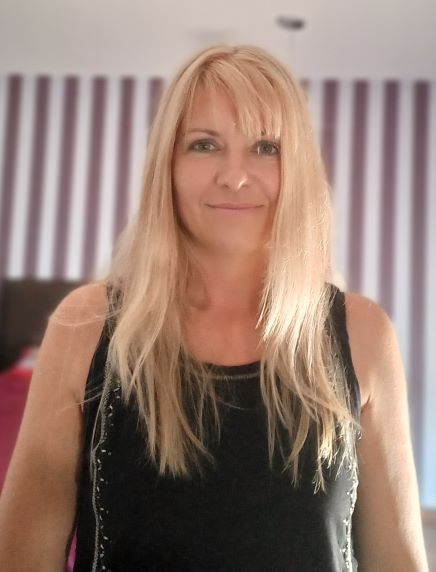
Tutorial: “Redes Neuronales: conceptos básicos y aplicaciones”
En este tutorial se pretende dar una visión introductoria al paradigma de las Redes Neuronales Artificiales (RNA). Se describirá brevemente el funcionamiento de las RNA y se mostrará cómo a través de la combinación de unidades simples de procesamiento (neuronas) interconectadas operando de forma paralela, se consigue resolver problemas complejos. Mediante la utilización de las notebooks de Colab, se desarrollarán aplicaciones que implementen RNA para el reconocimiento de formas o patrones, predicción y clasificación, utilizando el lenguaje Python. Como objetivo final se pretende proporcionar al participante, las bases que le permitan discernir cuándo y cómo poder aplicar este modelo computacional, entendiendo la “magia” que hay detrás de las RNA.Contact information
- Web: Personal page.
Maël Fabien & Juan Pablo Zuluaga
Ph.D. students at Idiap Research Institute, Switzerland
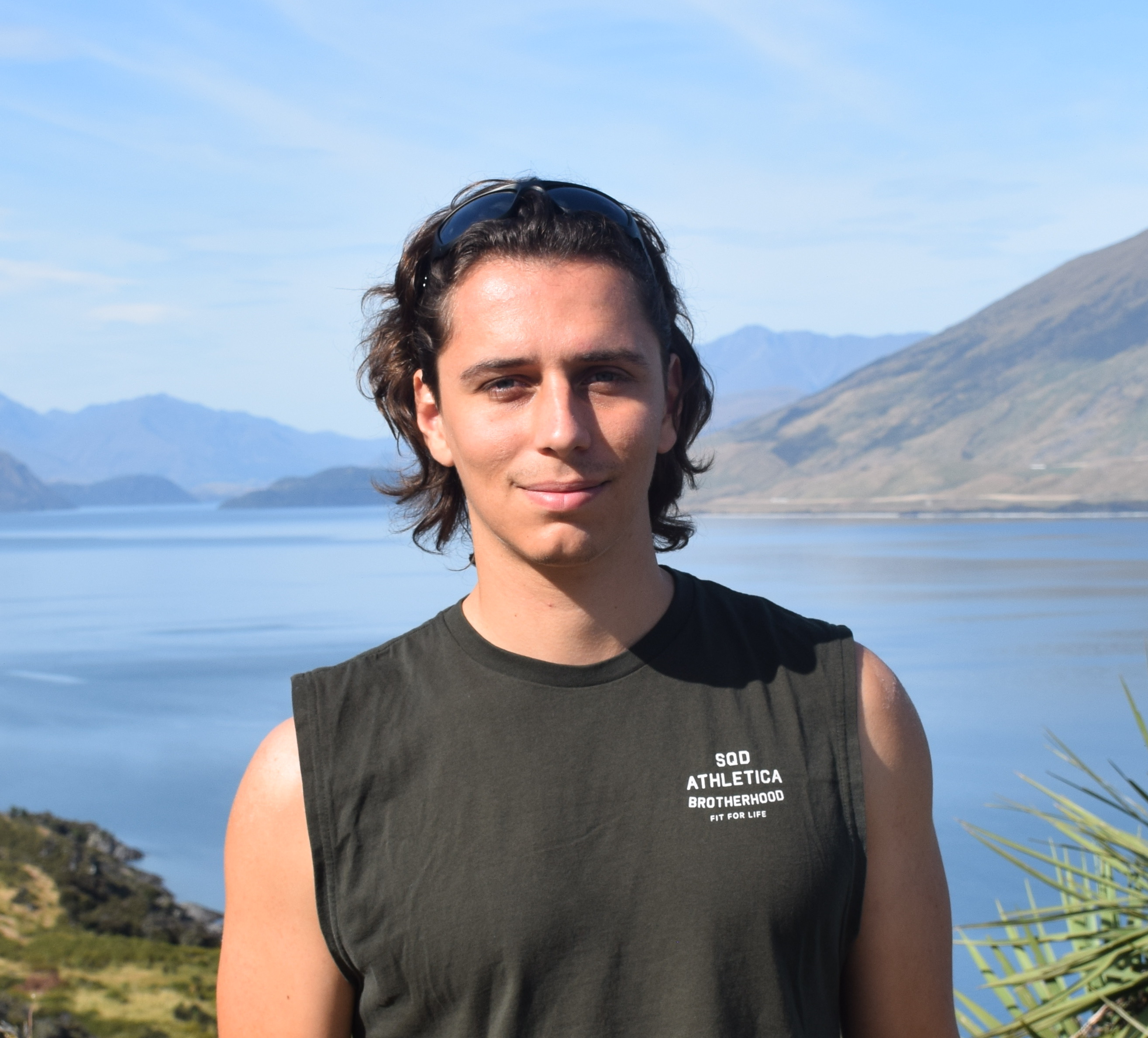
Maël is a Ph.D. student at Idiap Research Institute and EPFL, in speech processing, and applications related to combating organized crime. Maël has a background in Statistics and Data Science, and in widely interested in entrepreneurship and applied ML.
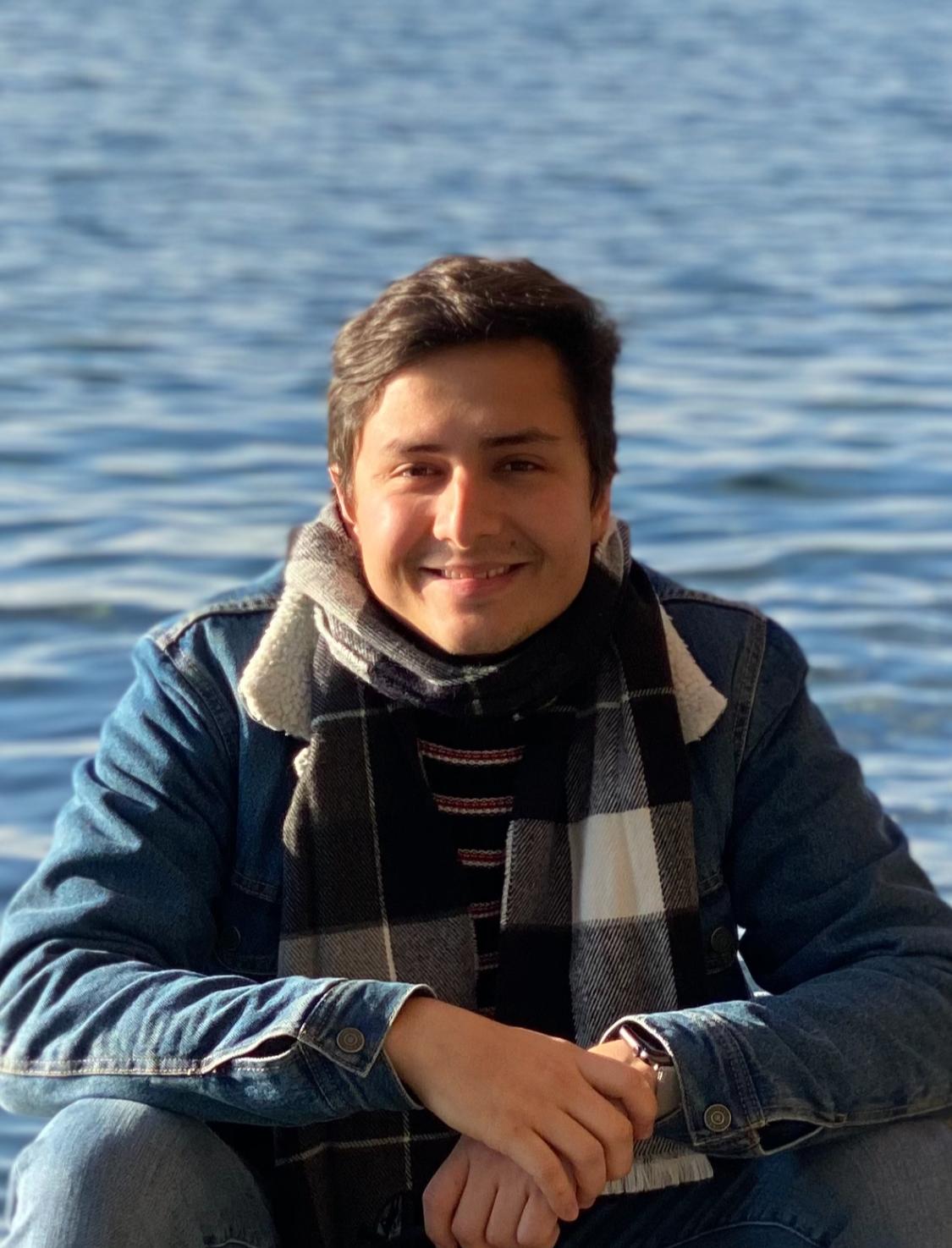
Tutorial: “An introduction to speech-based technologies for Natural Language Processing applications”
Since the last two decades, the amount of data generated and collected has grown exponentially, and especially through the rise of unstructured data such as images, videos or text. More recently, audio and speech data have gained a large interest, for example through voice assistants. Companies like Google, Facebook, Apple, and Amazon have shown an increasing interest in professionals with skills and tools for 'understanding' and 'transforming' the massive flow of speech data in relevant information. Some of the most important speech-based technologies are voice activity detection, speaker diarization and identification, and automatic speech recognition. These techologies are often used as an input to various NLP applications afterwards. This brief workshop will give you a set of basic tools for grasping the main aspects of speech-based technologies and how they can be implemented in real-life cases.Contact information
- LinkedIn: Maël's LinkedIn
- Blog: Maël's GitHub
- Twitter: Juan's Twitter
Panelists
Dr. Isabelle Augenstein
Professor at the University of Copenhagen
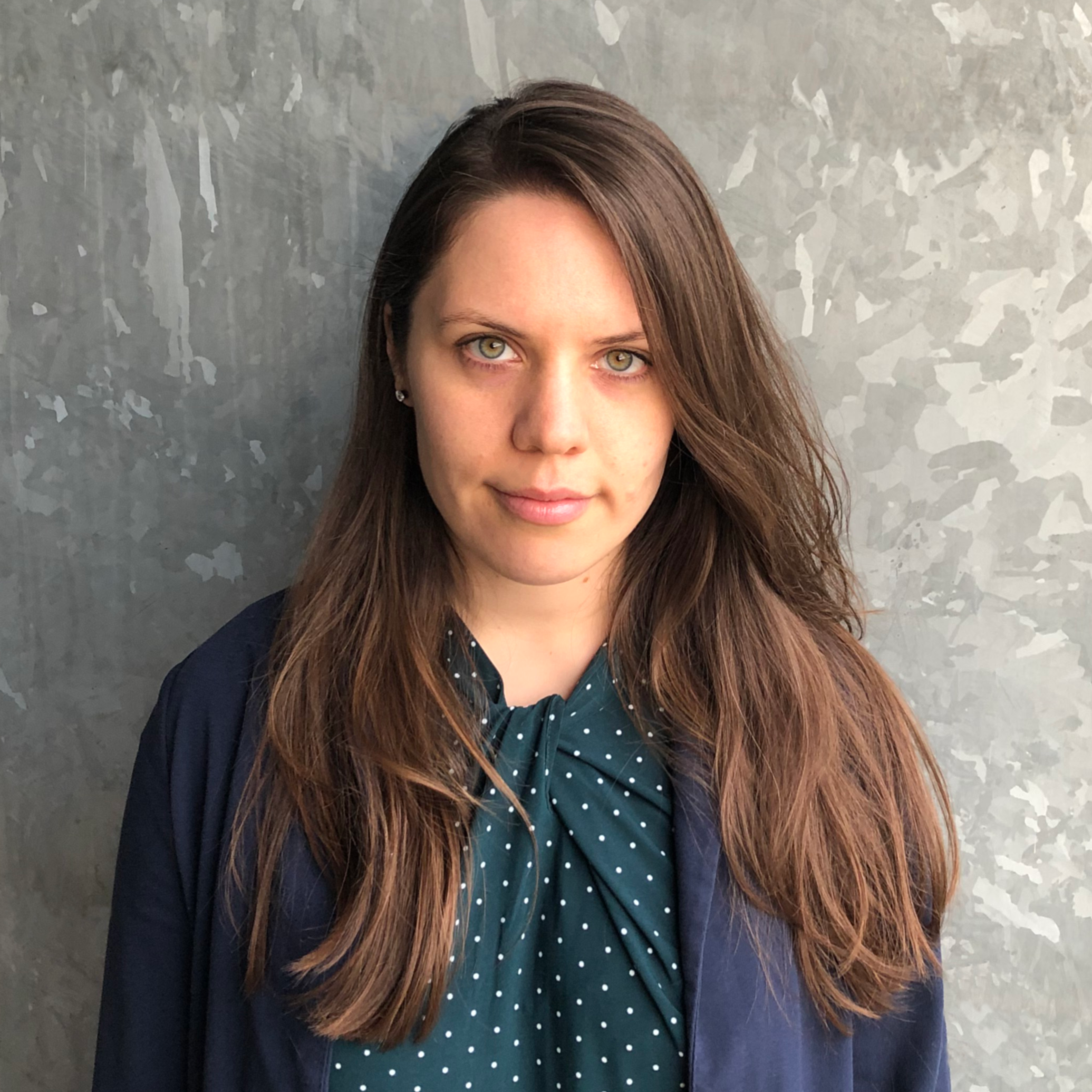
Dr. Luciana Benotti
Professor at the Universidad Nacional de Córdoba, Argentina
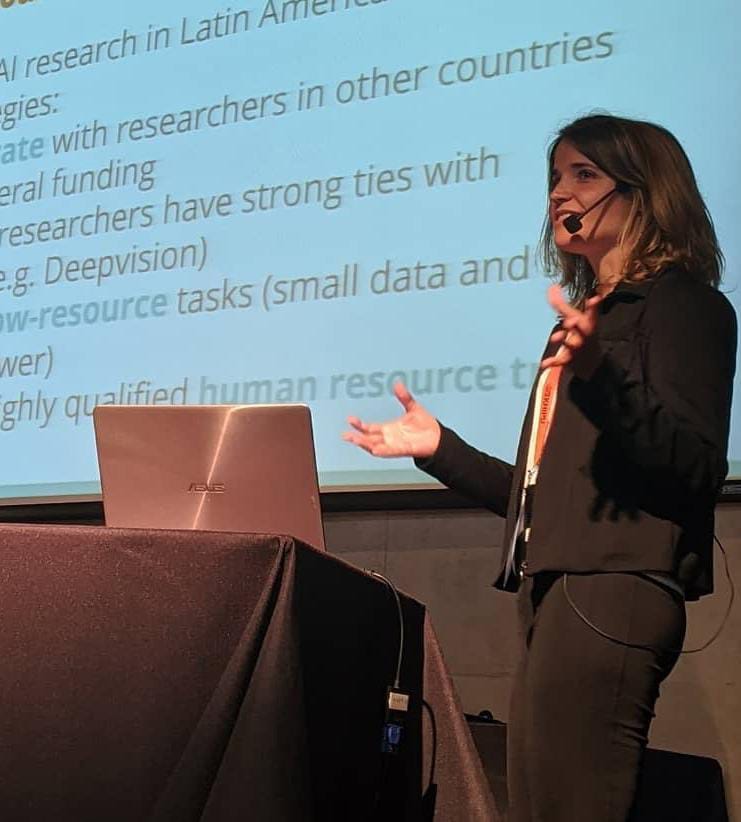
Dr. Ted Pedersen
Professor at the University of Minnesota, Duluth
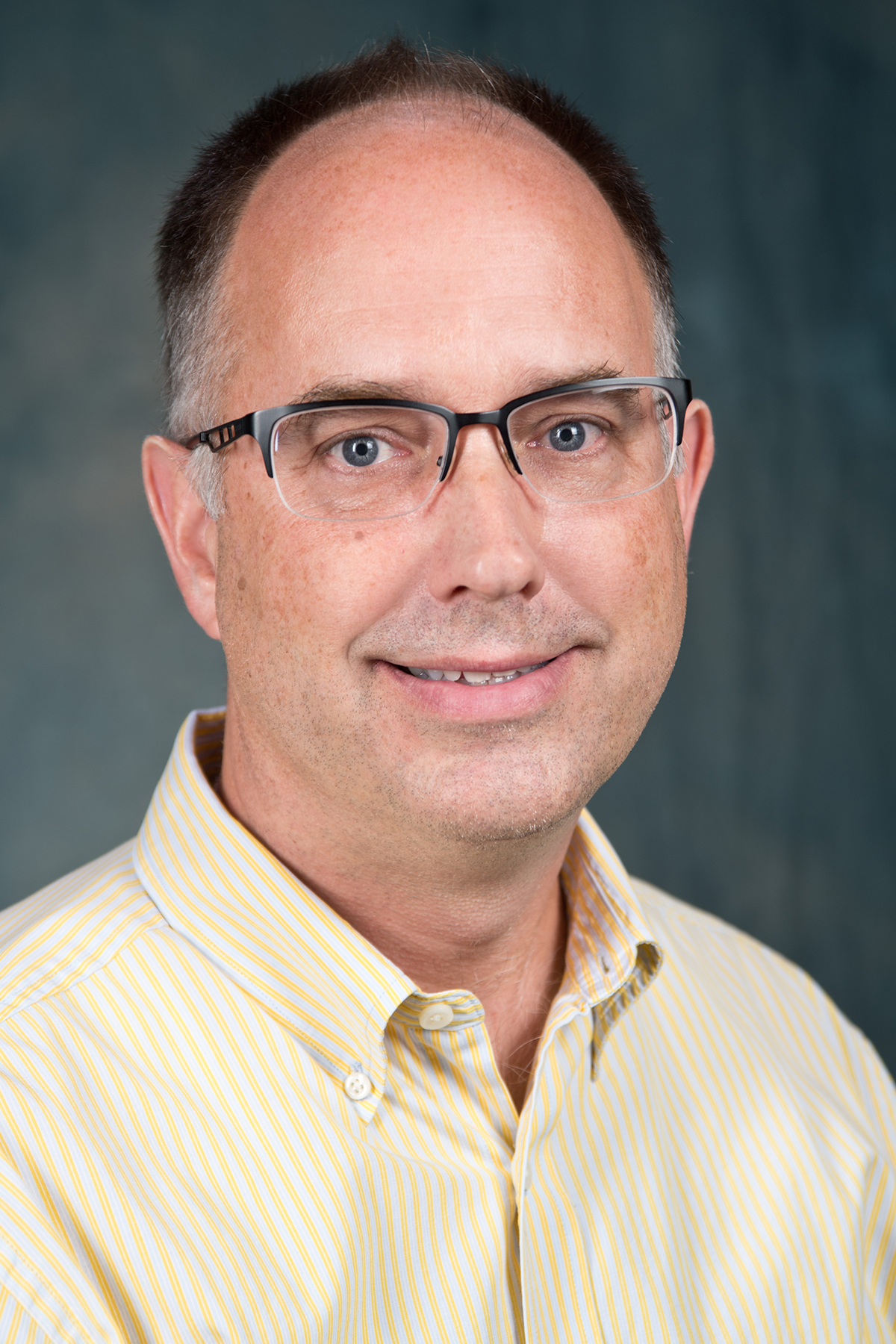
Contact information
- Web: Personal page.
Dr. Steven Bethard
Professor at the University of Arizona
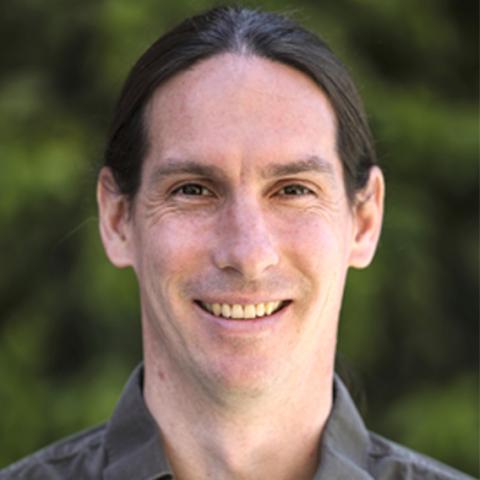
Contact information
- Web: Personal page.
Dr. Daisuke Kawahara
Professor at the Waseda University
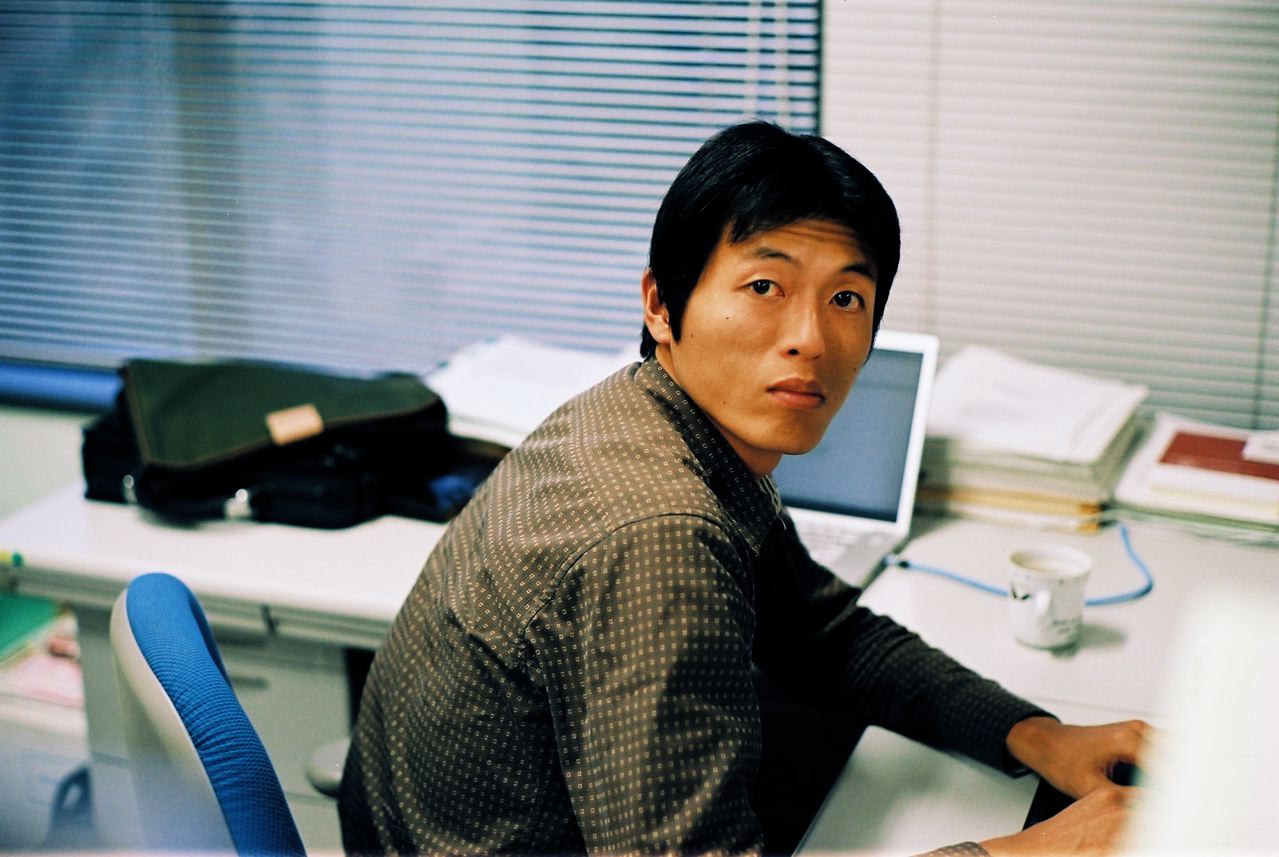
Dr. Francisco (Paco) Guzmán
Researcher at Facebook
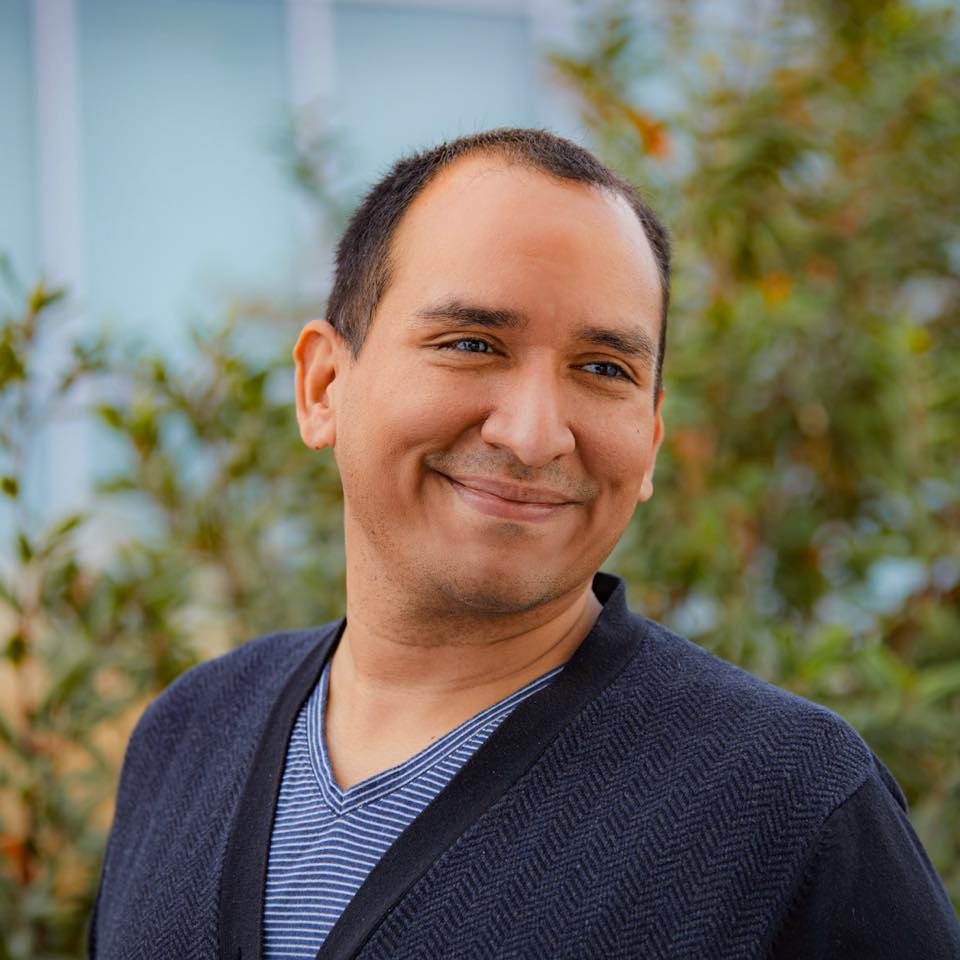
Dr. Gabriel Infante-Lopez
Principal Data Science at Proofpoint Inc.
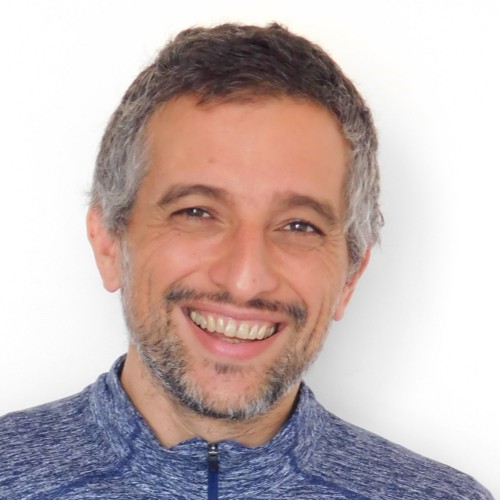